
Partners: |
 |
WORKSHOP INSTRUCTORS |
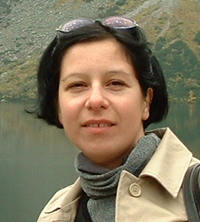 |
Workshop Lead: Dr. L. Monika Moskal is an AssociateProfessor of Remote Sensing at the University of Washington (UW), College of the Environment, School of Environmental and Forest Sciences & Precision Forestry Cooperative, where she directs the Remote Sensing and Geospatial Analysis Laboratory (RSGAL) |
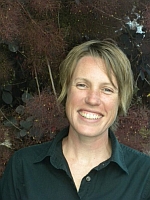 |
Workshop Instructor: Dr. Diane Styers was a Post-doctoral Research Associate who joined RSGAL in January 2010 till July 2011. Currently, Dr. Styers is an Assistant Professor of Remote Sensing in the Department of Geosciences and Natural Resources at Western Carolina University, located in Cullowhee, NC. |
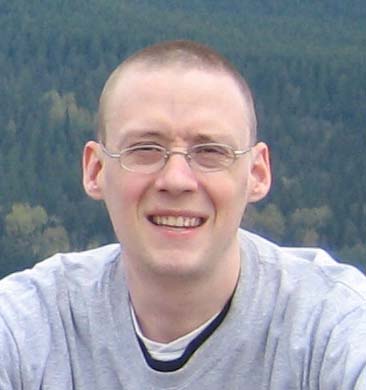 |
Workshop Graduate Research Assistant: Justin Kirsch is a MS student who joined us in 2010 from Evergreen State College where he worked under Dr. Dylan Fischer participating in monitoring of the Evergreen Ecological Observation Network. |
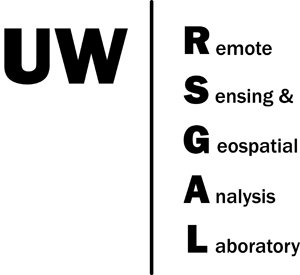 |
Workshop Volunteers: Matt Dressler (workshop tester); Meghan Halabisky, Alexandra Kazakova, David Stephens, Dr. Jeffery Richardson and Dr. Guang Zheng. |
NEWS |
- Trial workshops: Feb 15 & 28
- Workshop @ UW: March 3, 2011 -- this workshop is now full
- Online version will be available by June 15, 2011
- NEW: LiDAR workshop
|
|
- WHY GEOSPATIAL CANOPY ASSESSMENT?
- WORKSHOP
- DATA
- SOFTWARE
- READINGS
- OTHER
Remote sensing technologies can provide a means to explore a variety of continuous environmental variables over large areas. Remote assessments are reasonably simple and can be conducted quickly, inexpensively, and without access or disturbance issues encountered in ground-based data collections. These assessments provide a means to measure and monitor complex urban environments, and their dynamic ecologies.
For instance, canopy cover surveys and forest pattern metrics are useful to help a city quantify current tree cover status (Hunsinger & Moskal, 2005), determine the locations and drivers of canopy loss or gain (Turner & Gardner 1991), and monitor these trends in over time (Moskal et al. 2004). These data can then be used to establish tree protection requirements for new developments, assist with urban forest health management, and determine target areas for planting projects. Remote sensing techniques can be applied to the analysis of many other environmental and human dynamics within urban systems to aid in sustainable planning and management of these areas.
Traditional remote sensing techniques are not generally appropriate for assessing complex scenes like that of urban areas. Though historically used for earth observation, the spatial resolution of Landsat imagery (30 meters) limits the ability to map small features found within urban areas. In such cases, aerial imagery is generally preferred.
A relatively new method, object-based image analysis (GOBIA), sometimes referred to as feature extraction or object-based remote sensing, allows for use of additional variables such as texture, shape, and context to segment and classify image features (Hay & Castila 2008). This can both improve accuracy results and allow us to map very small urban features, such as mature individual trees or small clusters of shrubs (Moskal et al. 2011). |
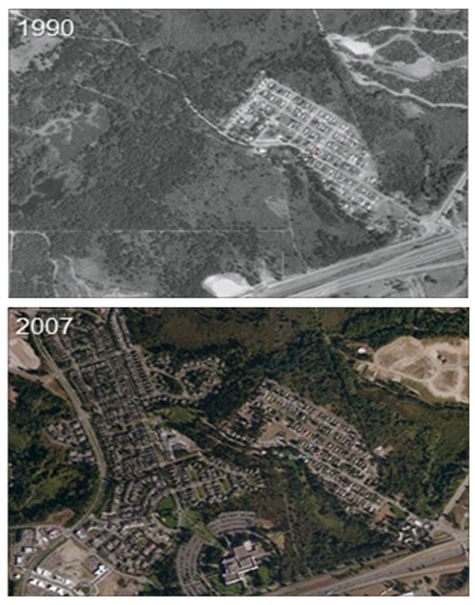 |
Figure 1. Aerial photography depicting land conversion from 1990-2007, DuPont, WA. |
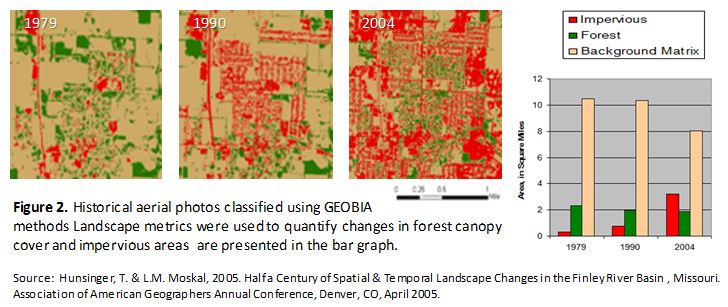 |
Although historical aerial photography has been available for over 60 years, due to improvements in image processing tools, it is just now begin to rapidly evolve as a management tool (Morgan et al. 2010). Land cover and land use classes, such as forest canopy and impervious areas (Figure 2) can be automatically classified and extracted from high resolution aerial and satellite imagery using new Object Based Image Analysis (OBIA) techniques (Hey and Castilia 2008). |
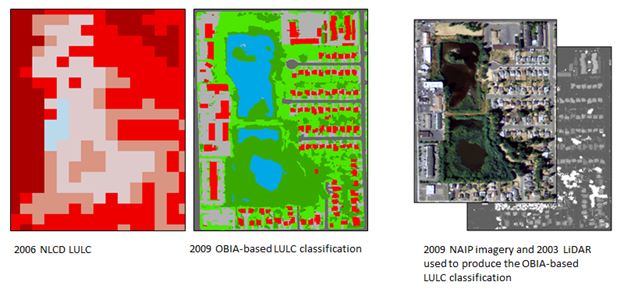 |
Figure 3. Comparing 2006 NLCD LULC to the 2009 OBIA-based LULC: The red in the 2006 NLCD data represent impervious surfaces, blue is water; note that no green (canopy) is summarized in this classification. The OBIA-based classification shows fine features such as canopy (green), buildings (red), impervious (gray) and water (blue). |
This workshop was designed by Dr. Moskal's UW Remote Sensing and Geospatial Analysis Laboratory with support from the following partners: The City of Tacoma, WA Department of Natural Resources, USDA Forest Service Pacific Northwest Research Station, Green Cities Research Alliance and the University of Washington Precision Forestry Cooperative.
The main purpose of this project is to provide guided analytical training to urban foresters, land managers, and city planners in an innovative technique to quantify tree canopy cover using high-resolution aerial imagery, calculate forest change metrics, and select sampling sites for ground-based tree inventories.
The project was funded by the 2010 DNR Urban and Community Forest Grants through a grant provided to the University of Washington (PIs Dr. L. Monika Moskal and Dr. Diane Styers).
The workshop promotes the use of publicly available remotely sensed data including the National Agricultural Imagery Program (NAIP) imagery and Puget Sound LiDAR Consortium data. The method of land use land cover (LULC) classification taught was the Object Based Image Analysis (OBIA) approach using the free SPRING software, and described in more detailing Appendix A; as this method is most suitable when using hyperspatial data such as 1m and sub-meter pixel imagery and LiDAR. The method is also suitable for extracting high detailed canopy cover, especially in heterogeneous areas such as urban environments where 30 m pixel data does not capture the fine spatial transitions in LULC. This workshop filled a need for city and county personnel in the state of Washington, who may not otherwise be able to afford training in the use of these new technologies in order to protect natural resources in their communities.
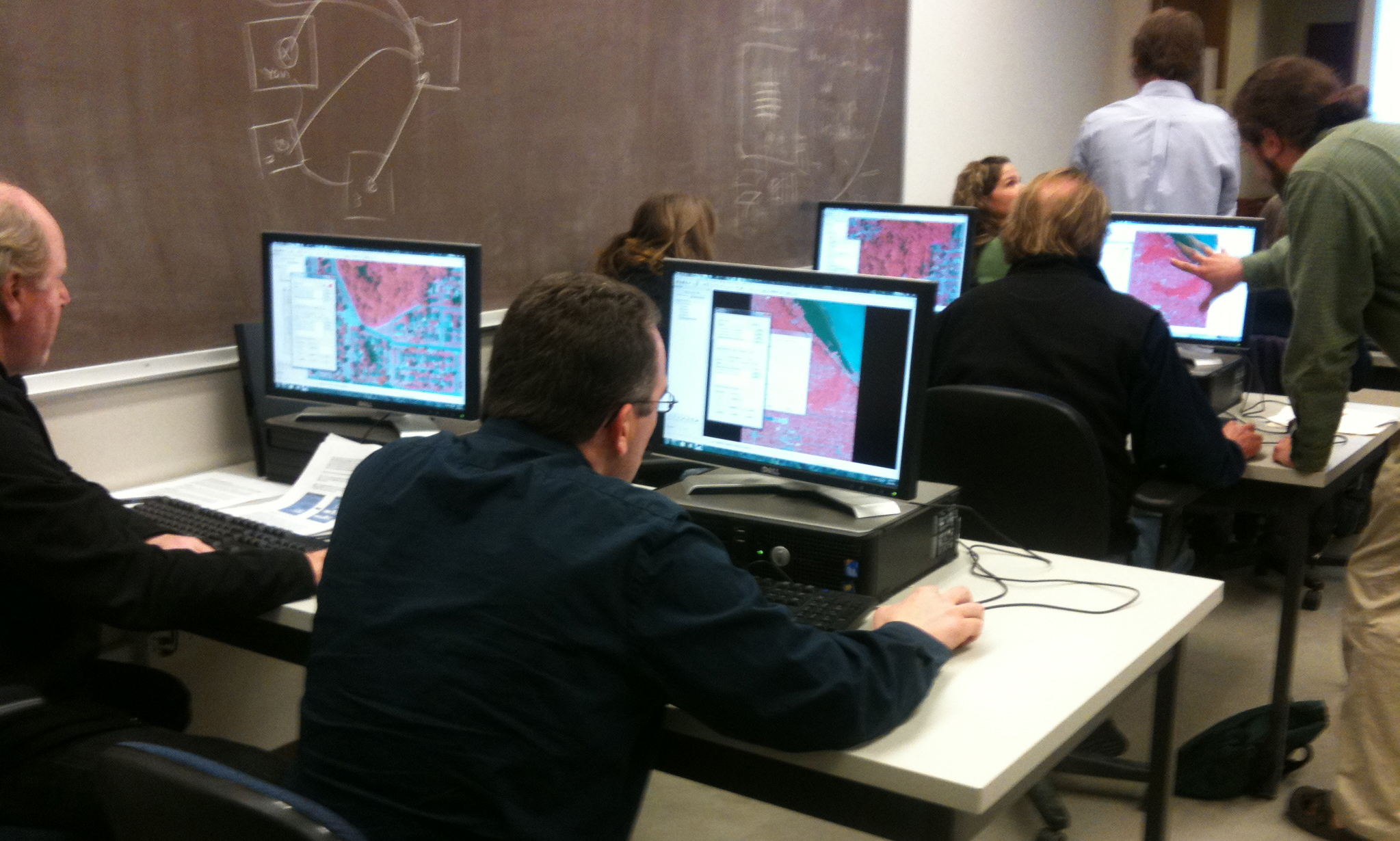 |
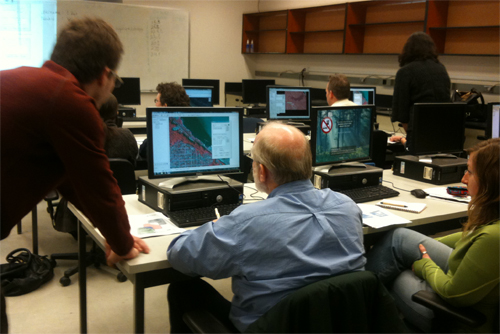 |
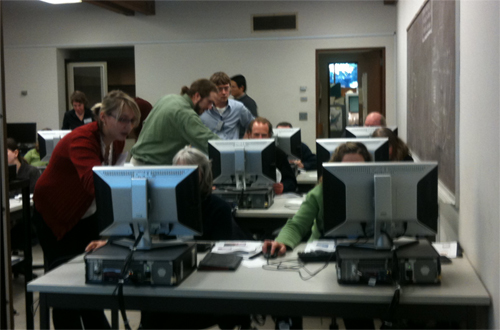 |
Photos from the 2011 workshop hosted by UW RSGAL |
To help you get started learning how to use OBIA for canopy cover assessments we provide a subset of the Tacoma data for you to work with. You can compare your final results to our map created using the Definiens eCognition software. We produced a full Land Use/Land Cover (LULC) map using the same 2009 NAIP imagery and LiDAR data layers; the accuracy of this approach is discussed in the project report.

2009 NAIP Imagery and LiDAR OBIA-based LULC for Tacoma, WA |
Our goal is to promote the use of open source or free software in all remote sensing projects. Thus, we used the SPRING software as the foundation of the workshop. It's a full suite of remote sensing analysis including pixel based approach and the Object Based Image Analysis (OBIA) approach. It's free and the code for it will eventually be released so you can modify it. You can also learn how to work with the software through the ESRM 430 Hyperspatial Remote Sensing Course taught at UW by Dr. Moskal.
If you plan to use LiDAR as a data layer to your analysis, you might have to pre-process the data to ground and canopy models, but don't worry you can do so with the FUSION software developed by the USDA Forest Service Pacific Northwest Research Station and it's free for non-commercial applications. You can also learn how to work with the software from our UW RSGAL LiDAR Workshop and the ESRM 430 Hyperspatial Remote Sensing Course taught at UW by Dr. Moskal. If you are looking for LiDAR data visit the Integrated Urban Forest Assessment Geoportal.
If you plan to use a non-georeferenced data source in your analysis, such as aerial photographs acquired form a kite or balloon mounted sensor, the free MapKnitter software will allow you to stitch and cover your image to a GeoTIFF
Finally, if you are thinking of acquiring your own imagery check out Grassroots Mapping.
Free Canopy Assessment Mapping Software List:
|
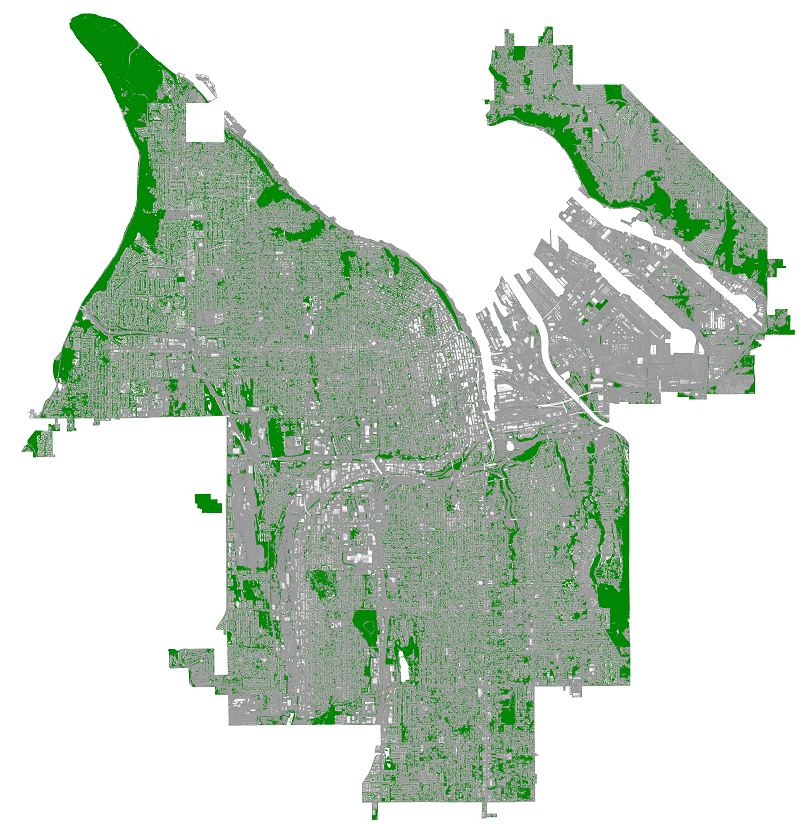
2009 NAIP Imagery and LiDAR OBIA-based Canopy Cover for Tacoma, WA |
We invite you to share your experiences, comments and suggestions regarding the workbook on the discussion board. Useful resources, links can also be posted on this board. We post major project updates to the board.
Other Links
Results from a landscape change geovisualization projects conducted by Dr. Moskal's |
|
|
Near the City of Ozark, MO in 1972 |
Near the City of Ozark, MO in 2000 |
|